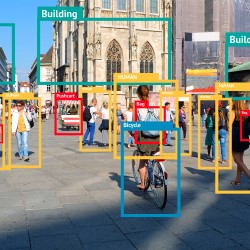
Real-time object detection first came on the scene at the dawn of the new millennium with the most basic visual object detection that identified human faces created by Paul Viola and Michael Jones. Fast-forward two decades, object detection has gone from rudimentary to highly sophisticated thanks to computer vision enhancements and deep learning improvements. In the new area of deep learning computer vision, which started around 2014, Regions with CNN features (RCNN) were created, setting the new standard for sophisticated object detection. This new framework allowed for technology to extract features from different regions in an image, bringing them together for enhanced classification.
Applications of Real-Time Object Detection
Now, real-time object detection is applicable in various cases, from enhanced safety and security in public transit systems to efficient automation in smart home products. Here’s a quick look at industries heavily utilizing real-time object detection.
- Surveillance and Security — Real-time object detection can quickly identify suspicious activity or objects in airports, subway systems, sports arenas, and other public gathering areas.
- Transportation — As autonomous vehicles become more of a reality, real-time object detection helps them avoid collisions and pedestrians and make rapid intelligent driving decisions.
- Healthcare — Inventory monitoring, patient monitoring, and activity detection help providers bring higher-quality care and improved patient safety.
There are even more applications across manufacturing, retail, gaming, and agriculture. However, with all these highly useful ways to leverage real-time object detection, unique challenges around run time and the heavy computing load of these detection systems make it difficult for proper adoption, standardization, and utilization.
The Outlook
Even while more industries and applications look to sophisticated deep learning computer vision technology, it’s a time and labor-intensive process. With the market poised to hit a valuation of $41.11 billion by 2030 with explosive growth over the next decade, necessary advancements need to be quickly made around processing and accuracy. A Region Proposal Network (RPN) enters as a potential solution for high-quality region proposals that feed into faster R-CNN. Take a look at this idea proposed in “Faster R-CNN: Towards Real-Time Object Detection with Region Proposal Networks” for creating a fully convolutional network.
Download the Full Study