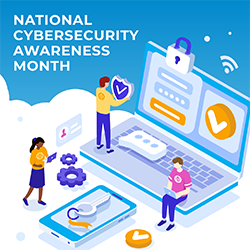
The applications of Machine Learning techniques have exploded in recent years due in part to advances in data science and high-performance computing. For example, it is now possible to collect, store, manipulate, analyze and retain massive amounts of data, and therefore the Machine Learning systems are now able to learn patterns from this data and make useful predictions.
These systems are being used in practical applications in various fields such as medicine, finance, marketing, defense, and manufacturing. They are also being applied to solve cyber security problems such as malware analysis and insider threat detection.
Related: During Cybersecurity Month 2019, we offer you the free Oct. 23 webinar “Lessons Learned from Snowden’s former NSA boss: Strategies to protect your data.” Sign up now and get bonus content of three exclusive articles!
However, there is also a major concern that the machine learning techniques themselves could be attacked by adversaries including nation states and industry competitors. For example, an adversary may learn the machine learning model used and subsequently modify its behavior and even prevent the adversary from getting caught. The anti-malware products may be inadequate to detect such attacks.
When this happens the machine learning models could produce incorrect results. Imagine if a machine learning system gives advice to a physician to give triple the dosage that is needed for a patient for diabetes? Therefore, the machine learning models have to anticipate the ways in which the malware may modify itself.
This may result in adapting the models. After a period of time, the malware will catch on to the adaptive behavior of the machine learning models and attempt to thwart the models. This type of game playing may go on until one party wins. Therefore, our challenge is to develop solutions to handle such attacks by the adversary.
Such solutions have come to be known as adversarial machine learning. The question is, what is the utility of the machine learning techniques when they are subject to adversarial attacks? This is one of the more challenging problems faced today by cyber security researchers and practitioners.
Bhavani Thuraisingham is the Founders Chair Professor of Computer Science and the Executive Director of the Cyber Security Research and Education Institute at The University of Texas at Dallas. She is a Fellow of IEEE and ACM.