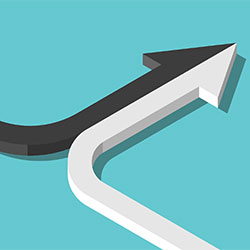
For as long as there have been corporations, there have been corporate mergers. The same goes for other types of businesses of all sizes and varieties. Already this year, there have been more than $2 trillion worth of mergers worldwide – and more are likely on the way. In the past, joining two separate business entities together required careful planning and de-duplication of operations, elimination of redundant staffing, and optimization of the resulting entity. In the past several years, however, a new wrinkle has made such mergers more complex than ever before – the need to figure out how to combine separate and often complex data operations.
The size of the challenge that can pose is enormous. As more companies invest larger sums into their big data and analytics functions, they’re creating mountains of information that can present a real obstacle to creating a single unified approach to the task. It isn’t a simple matter of sorting everything out, either. There’s also the matter of safeguarding the existing data, meeting any relevant regulatory requirements, and figuring out how to merge whatever data mining and processing operations exist in the original organizations.
The problem with that is the fact that it’s uncharted territory. Businesses are only now coming to terms with the scale of the data they’re in possession of, and most are still in the early stages of mastering their own processes. A sudden influx of unfamiliar procedures and systems can wreak havoc on everyone involved. There are, however, a few strategies that businesses can use to handle the merging of data operations. Each has its own strengths and weaknesses, and each is suited for a specific circumstance. Here’s what they are.
Bridging the Divide
Rather than beginning the process by putting all of the two businesses’ data assets into a single system, it’s sometimes preferable to construct some links between the existing systems and allow them to continue to function independently. There are a variety of firms that specialize in the process of data mapping, which consists of building a coherent connection between whatever systems are presently in use.
Adeptia, for example, operates a platform that was built for this express purpose. It’s typically used by businesses that need to onboard partner organizations, but the methodology works just as well as the first step toward the permanent integration of two businesses into one. With it, two companies can introduce automated processes that enable seamless data flow between disparate systems. At that point, the merged businesses can then focus on de-duplication tasks before deciding how to proceed next. Taking this approach is useful for firms that are merging distinct lines of business – as there may be no real need to create a unified operation.
The downside, of course, is that this can harm efficiency in the long run if there’s too much overlap between the two remaining units. It also doesn’t lend itself well to data standardization, which can be a stumbling block in the future when the merged business attempts to scale up. Regardless, it’s a viable option when used the right way.
Constructing a New, Purpose-Built System
In situations when it’s apparent that there will be large areas of overlap in the existing data operations, it can be beneficial to take a start-from-scratch approach. Doing so requires the establishment of a single new entity that will control the merged business’s data, and having them undertake a top-down review of existing processes and systems. Once they have a firm grasp on the size, scope, and types of data they have to deal with, they can establish a whole new master process to go forward with.
The steps to doing this should be almost identical to what each business did when establishing their current data operations, but with some new variables added to the mix. The upside of this approach is that it will result in a single data operation that’s free from any inefficiency that may have come along with the original infrastructures. It also forces the combined company to reckon with whatever data protection or regulatory issues may apply early on in the process, so they won’t cause problems later on. The downside, of course, is that it can (aside from any reuse of existing systems) result in throwing away the capital investments the two companies had made to get to the point they were at pre-merger.
Choosing a Superior
For better or worse, in most mergers, there will be one business that’s larger and more established than the other. In that scenario, the preferred methodology is to make the decision to roll the smaller company’s data operations into the larger one, and then eliminate the smaller company’s data unit. In most cases, this is the path that businesses choose to follow in a merger. If the larger business’s data systems are robust and adaptable, this is a reasonable and cost-effective approach.
When they aren’t, however, the results can be catastrophic. First, if every possible issue isn’t resolved before any data is migrated to the larger company’s systems, it can lead to an explosion of costs, or worse – cause the whole operation to grind to a halt. Second, the data teams from the smaller company might face irrelevance and leave the organization, robbing the new entity of valuable know-how and experience. In fact, this is a situation that plays out with disturbing frequency when mergers happen. If left unchecked, the new combined company might not be able to execute whatever data strategy they’re tasked with when the dust settles.
The Bottom Line
No matter the route chosen, there’s no single sure path to putting the data operations of two distinct organizations together without some sacrifices or short-term pain. The right way to go about it depends on innumerable factors, and there is a high potential for unexpected issues to complicate whatever plan is used. As the merging of businesses with complex data operations continues to occur, there’s a good chance that some kind of consensus about which method is the least disruptive will emerge. Until then, companies facing the difficult process of combining complex, often relatively new data and analytics operations will have to choose the path they believe suits their situation best – and then do whatever is necessary to make it work. That, of course, comes with the territory for companies on the leading edge of the big data and analytics revolution.