For this ComputingEdge issue, we asked Naren Ramakrishnan—professor of engineering and director of the Discovery Analytics Center at Virginia Tech University—about big-data career opportunities. Ramakrishnan’s research interests include mining scientific datasets in domains such as systems biology, neuroscience, sustainability, and intelligence analysis. He was a co-guest editor for Computer’s April 2016 special issue on big data.
ComputingEdge: What careers in big data will see the most growth in the next several years?
Ramakrishnan: With this space maturing, more than seven of 10 organizations in the US are expected to have an in-house data science team by the end of this year. Demand for data scientists will grow in technical areas like deep learning, as well as in fields such as healthcare, the Internet of Things economy, finance, manufacturing, educational innovation, sustainability, and forecasting. You can keep track of what’s going on in data science forums such as KDnuggets (www.kdnuggets.com).
ComputingEdge: What would you tell college students to give them an advantage over the competition?
Ramakrishnan: Remember those courses you thought were boring and had nothing to do with real-life, like differential calculus, Bayesian statistics, graph theory, and linear algebra? They are the foundations of data science today! So spend time honing your fundamentals in college. It will prepare you for advanced courses and careers in areas such as deep learning, computer vision, and sensor mining. It’s also important to develop a portfolio of your data-analytics and visualization code, perhaps hosted on a GitHub page. Many prospective employers want to see examples of your big-data and data-analytics skills.
ComputingEdge: What should applicants keep in mind when applying for big data jobs?
Ramakrishnan: Just as data-science applications are varied, so are the job titles, responsibilities, and expectations. Find out how data science fits into a potential employer’s organizational structure. Do they have a CDO (chief data officer) or CIO (chief information officer)? Does data science play a supporting role or is it an integral part of the way they do business? How many business units within the organization rely on data science? These questions are important to understand how you will fit within the organization and how the organization will fit within your career objectives.
ComputingEdge: How can new hires make the strongest impression in a new position?
Ramakrishnan: There are significant open source software and frameworks for data analytics and visualization. Get familiar with these tools before joining your company. Once you start, you should be able to leverage this background to rapidly analyze data, perform exploratory or predictive analysis, and develop visual dashboards for demonstration to managers. Nothing conveys a stronger impression than a person who is able to complete the loop from data to insights to decisions. This will position you for interesting assignments and professional growth.
ComputingEdge: Name one critical mistake for young graduates to avoid when starting their careers?
Ramakrishnan: One common error is not taking the time to understand how data permeates your organization—for example, how data is produced and collected, who makes decisions based on data analytics, and what types of decisions these are. Understanding a bit about the larger picture will help you be a more effective data scientist. Get out of your comfort zone and speak to nontechnical professionals to understand the domain. Then when you speak as a data scientist, people will take your conclusions more seriously.
ComputingEdge: Do you have any learning experiences you could share that could benefit those just starting out in their careers?
Ramakrishnan: Data science is so pervasive today that it provides insights into situations and events in entirely unexpected ways. My favorite example has to do with the OpenTable website (www.opentable.com), which lets users make restaurant reservations in many cities worldwide. The site lets analysts download aggregate reservation data for specific cities on a daily—and even hourly—basis. In analyzing this data, my collaborators and I found that spikes in cancellations can correspond to health events, such as the flu season’s early onset or a food-contamination episode. The temporal profile of restaurant availability can help forecast flu seasonality. The lesson to take away from this is that data will show up in surprising places and can provide valuable insights if we just know where to look and how to relate disparate datasets. One should consciously think about data they encounter in real life and study its implications.
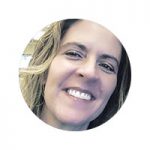
About Lori Cameron
ComputingEdge’s Lori Cameron interviewed Ramakrishnan for this article. Contact her at l.cameron@computer.org if you would like to contribute to a future ComputingEdge article on computing careers. Contact Ramakrishnan at naren@cs.vt.edu.